The fractional knapsack problem involves selecting items to fill a knapsack of limited capacity (M=20 in this case) in such a way as to maximize the total value. Here's how to solve it: Sort the items in decreasing order of their value per unit weight (i.e. Pi/Wi). Item 1: 3/5 = 0.6 Item 2: 10/13 =Read more
The fractional knapsack problem involves selecting items to fill a knapsack of limited capacity (M=20 in this case) in such a way as to maximize the total value.
Here’s how to solve it:
Sort the items in decreasing order of their value per unit weight (i.e. Pi/Wi).
Item 1: 3/5 = 0.6
Item 2: 10/13 = 0.769
Item 3: 15/12 = 1.25
Item 4: 5/8 = 0.625
Take the items in the order of the sorted list until the knapsack is full. If a complete item cannot fit, take a fraction of it.
Item 3 (15/12) can fit completely, so add it to the knapsack and reduce the capacity to 20-12=8.
Item 2 (10/13) can fit completely, so add it to the knapsack and reduce the capacity to 8-13= -5.
Item 1 (3/5) cannot fit completely, so add 3/5 of it to the knapsack and reduce the capacity to 0.
Item 4 (5/8) is not considered since the knapsack is full.
The total value of the items in the knapsack is 15 + 10 + (3/5)*3 = 22.
So the optimal solution is to choose items 3, 2 and a fraction of item 1, with a total value of 22.
The time complexity of the subset sum problem using dynamic programming technique is O(nW), where n is the number of items and W is the target sum. The space complexity is O(nW). The most time-consuming part of the algorithm is the bottom-up dynamic programming loop, where each cell in the n x W matRead more
The time complexity of the subset sum problem using dynamic programming technique is O(nW), where n is the number of items and W is the target sum. The space complexity is O(nW).
The most time-consuming part of the algorithm is the bottom-up dynamic programming loop, where each cell in the n x W matrix is filled in. This loop takes O(nW) time in total.
The most space-consuming part of the algorithm is the n x W matrix itself, which requires O(nW) space to store the results of the dynamic programming.
Overall, both the time and space complexity are directly proportional to the size of the n x W matrix, making the dynamic programming solution to the subset sum problem an exponential-time algorithm.
Total number of characters in the message = 100. Each character takes 1 byte. So total number of bits needed = 800. After Huffman Coding, the characters can be represented with: f: 0 c: 100 d: 101 a: 1100 b: 1101 e: 111 Total number of bits needed = 224 Hence, number of bits saved = 800 - 224 = 576Read more
Total number of characters in the message = 100.
Each character takes 1 byte. So total number of bits needed = 800.
After Huffman Coding, the characters can be represented with:
f: 0
c: 100
d: 101
a: 1100
b: 1101
e: 111
Total number of bits needed = 224
Hence, number of bits saved = 800 - 224 = 576
Huffman Coding | Greedy Algo-3 - GeeksforGeeks
Solve using fractional knapsack: M=20, n=4 P= (3, 10, 15, …
The fractional knapsack problem involves selecting items to fill a knapsack of limited capacity (M=20 in this case) in such a way as to maximize the total value. Here's how to solve it: Sort the items in decreasing order of their value per unit weight (i.e. Pi/Wi). Item 1: 3/5 = 0.6 Item 2: 10/13 =Read more
The fractional knapsack problem involves selecting items to fill a knapsack of limited capacity (M=20 in this case) in such a way as to maximize the total value.
Here’s how to solve it:
So the optimal solution is to choose items 3, 2 and a fraction of item 1, with a total value of 22.
See lessAnalyse the algorithm (in terms of both time and space) …
The time complexity of the subset sum problem using dynamic programming technique is O(nW), where n is the number of items and W is the target sum. The space complexity is O(nW). The most time-consuming part of the algorithm is the bottom-up dynamic programming loop, where each cell in the n x W matRead more
The time complexity of the subset sum problem using dynamic programming technique is O(nW), where n is the number of items and W is the target sum. The space complexity is O(nW).
The most time-consuming part of the algorithm is the bottom-up dynamic programming loop, where each cell in the n x W matrix is filled in. This loop takes O(nW) time in total.
The most space-consuming part of the algorithm is the n x W matrix itself, which requires O(nW) space to store the results of the dynamic programming.
Overall, both the time and space complexity are directly proportional to the size of the n x W matrix, making the dynamic programming solution to the subset sum problem an exponential-time algorithm.
See lessA networking company uses a compression technique to encode the …
Total number of characters in the message = 100. Each character takes 1 byte. So total number of bits needed = 800. After Huffman Coding, the characters can be represented with: f: 0 c: 100 d: 101 a: 1100 b: 1101 e: 111 Total number of bits needed = 224 Hence, number of bits saved = 800 - 224 = 576Read more
Write the features of dynamic programming
Your Answer is in this image.
Your Answer is in this image.
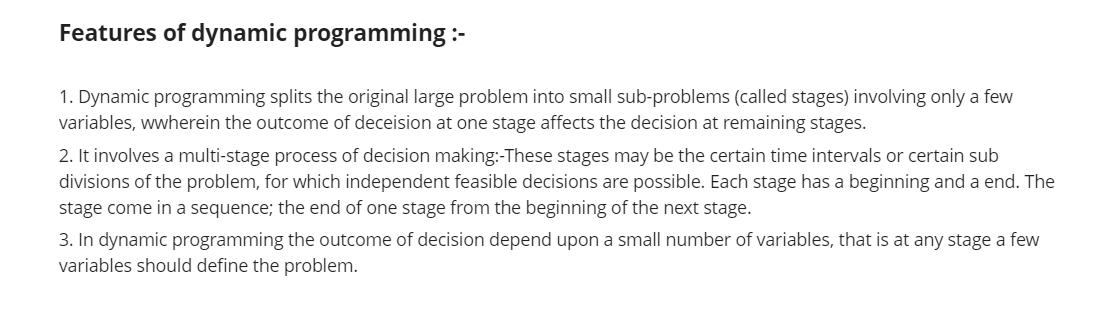
See less